The challenge
Chest X-rays are among the most frequently performed imaging studies worldwide. They are essential for diagnosing lung infections, chronic diseases, and other thoracic abnormalities. However, interpreting chest X-rays can be challenging due to the subtle nature of many findings, the variability between patients, and the high workload faced by radiologists. ML algorithms, especially deep learning models such as convolutional neural networks (CNNs), can support radiologists by accurately identifying disease markers, speeding up the diagnostic process, and reducing the risk of missed diagnoses.
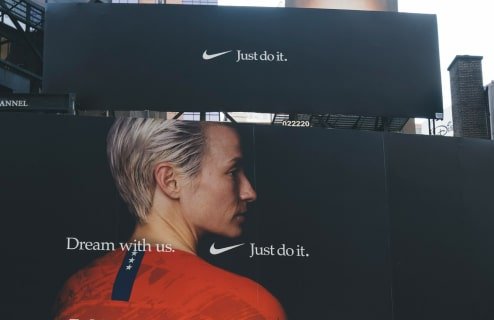
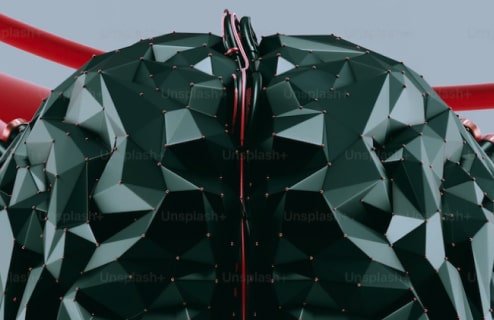
ML Applications in Chest X-Ray Imaging: Enhancing Disease Detection and Diagnostics
Machine Learning (ML) models are transforming chest X-ray diagnostics, aiding in the detection of conditions like pneumonia, tuberculosis, COVID-19, and other lung-related diseases. Key applications include:
1. Disease Detection in Chest X-Rays
ML models initially gained traction in pneumonia detection, a crucial application that set the stage for further developments. For example, CheXNet, a CNN-based model developed at Stanford, was trained on the NIH ChestX-ray14 dataset, which includes over 100,000 labeled X-ray images. CheXNet demonstrated diagnostic accuracy on par with expert radiologists, highlighting ML’s potential to enhance clinical outcomes. During the COVID-19 pandemic, similar ML models adapted to detect patterns associated with COVID-19, assisting in patient prioritization and severity assessment, particularly in resource-constrained environments.
2. Multi-Disease Detection
ML advancements now allow models to detect multiple thoracic diseases within a single X-ray image, covering conditions like pleural effusion, cardiomegaly, and lung nodules that can indicate early lung cancer. By employing multi-label classification techniques, these models provide radiologists with a comprehensive view, enabling quicker and more accurate diagnoses.
Advantages of ML in Chest X-Ray Analysis
The adoption of ML in chest X-ray analysis offers several benefits:
Enhanced Diagnostic Accuracy: ML models can identify subtle abnormalities, potentially reducing diagnostic errors. Studies show that these models often match or exceed the accuracy of experienced radiologists.
Improved Efficiency and Reduced Workload: With the large number of X-rays processed daily, ML can provide preliminary evaluations, highlight urgent cases, and allow radiologists to focus on more complex cases, which is especially helpful in fast-paced environments like emergency departments.
Broader Access to Quality Care: In rural or resource-limited settings, ML-powered diagnostic tools make timely and accurate X-ray interpretations possible, thus promoting equitable healthcare.
ML applications in chest X-ray imaging are advancing diagnostic accuracy, efficiency, and accessibility. By assisting radiologists with disease detection and case prioritization, ML holds substantial potential to improve patient care. While challenges remain, ongoing research and development are likely to make ML a vital component of radiology.
Challenges in ML-Based Chest X-Ray Diagnostics
Despite its promise, the integration of ML in chest X-ray imaging presents several challenges:
Data Quality and Annotation: ML models require large, expertly annotated datasets. This data is costly and time-intensive to collect, and differences in imaging protocols across facilities can complicate model training.
Model Interpretability and Clinical Trust: Many ML models function as “black boxes,” providing predictions without a clear explanation. This lack of transparency can reduce radiologists’ confidence in these tools.
Regulatory and Ethical Considerations: ML models in medical applications must meet stringent safety standards, which can slow down regulatory approvals. Additionally, addressing model biases related to factors like race or age is essential to ensure fair and unbiased diagnostics.
Case Study: CheXNet for Pneumonia Detection
CheXNet, a pioneering model from Stanford, exemplifies ML’s potential in chest X-ray diagnostics. Built with a 121-layer CNN architecture and trained on NIH’s extensive ChestX-ray14 dataset, CheXNet accurately detects pneumonia, performing comparably to expert radiologists. However, clinical deployment of CheXNet required addressing issues related to interpretability, regulatory standards, and consistency across diverse patient groups.
Future Prospects
ML in chest X-rays is poised to evolve, focusing on improved model transparency, robustness through federated learning (training on multiple decentralized datasets), and streamlined integration into clinical workflows. Furthermore, combining chest X-rays with additional patient data (multimodal ML) could enhance diagnostic accuracy, benefitting both patients and healthcare providers.
Precision in Diagnosis
Workflow Optimization
Expanded Access
Reliable Consistency